Back to Projects List
Automatic Segmentation of Teeth and Alveolar bone using MONAI Label
Key Investigators
- Daniel Palkovics (Semmelweis University, Budapest Hungary)
- Csaba Pinter (EBATINCA, Las Palmas de Gran Canaria, Spain)
- Andrés Diaz-Pinto (King’s College, NVidia)
Project Description
A three-dimensional visualization of dento-alveolar structures can enhance the surgical planning process, however currently there are no reliable fully automated segmentation methods available to acquire realistic 3D virtual models of teeth and alveolar bone. A time consuming semi-automatic method has previously been utilized for diagnostic purposes and surgical planning of regenerative-reconstructive surgical procedures in periodontology and oral surgery.
Objective
The aim of this project is to develop an automatic method utilizing MONAI Label to speed up segmentation process of dento-alveolar structures on cone-beam computed tomography datasets.
- Objective A. To develop a fast and relible segmentation method that is capable of the separate 3D reconstuction of teeth and alveolar bone on CBCT datasets of periodontally involved patients
Approach and Plan
- Try to create MONAILabel app for segmenting said structures
- Consult with the experts about the details
Progress and Next Steps
- Through varios conversations with especially Andrés (special thanks to him), we learned the following that will be useful for this project:
- It is possible to segment the teeth as one structure or per-tooth as well. In both cases we will need to use MultiLabelDeepEdit due to the presence of the alveolar bone structure
- It is possible to set up multi-stage inference in which the first stage can be preprocessing such as automatic cropping or removal of artifacts such as implants or bridges
- For initial experimentation a typical desktop GPU with 8GB can be used, however to achieve a well performing model it is recommended to have a professional one with >16GB memory
- A feasible option for this is to use an AWS instance
- This problem is different from the majority of segmentation tasks because missing structures are very commonplace (i.e. missing teeth especially for a patient who needs implant planning)
- The issue of the missing teeth needs to be carefully handled
- Use consistent numbering (see image below)
- Make sure the same teeth have the same label # (i.e. skip those that are not present)
- The way Slicer exports the segmentations does not fully support this use case due to the many missing structures
- Plan to improve segmentation export in Slicer
- Add option both in segmentation export widget and segmentation logic to use the current terminology context for generating the same label for each structure (see image below)
- Many details to figure out: How to handle modifiers and anatomic regions, What happens when there are more than 255 usable entries, etc. We will start simple.
- Create a custom terminology context for this use case (alveolar bone + the 32 teeth, see image below)
- Update the existing datasets to have the correct terminology of each structure and re-export the segmentations
- Create simple module for single-click batch export of the MRBs for DeepEdit usage (to make sure the segmentation extent is the same as the master volume extent and to use terminology-based label numbers)
- UPDATE: We will try first to do the export using a custom color table we create together with the terminology and use the existing feature that assigns labels based on a selected color table
Illustrations
Example segmentation:
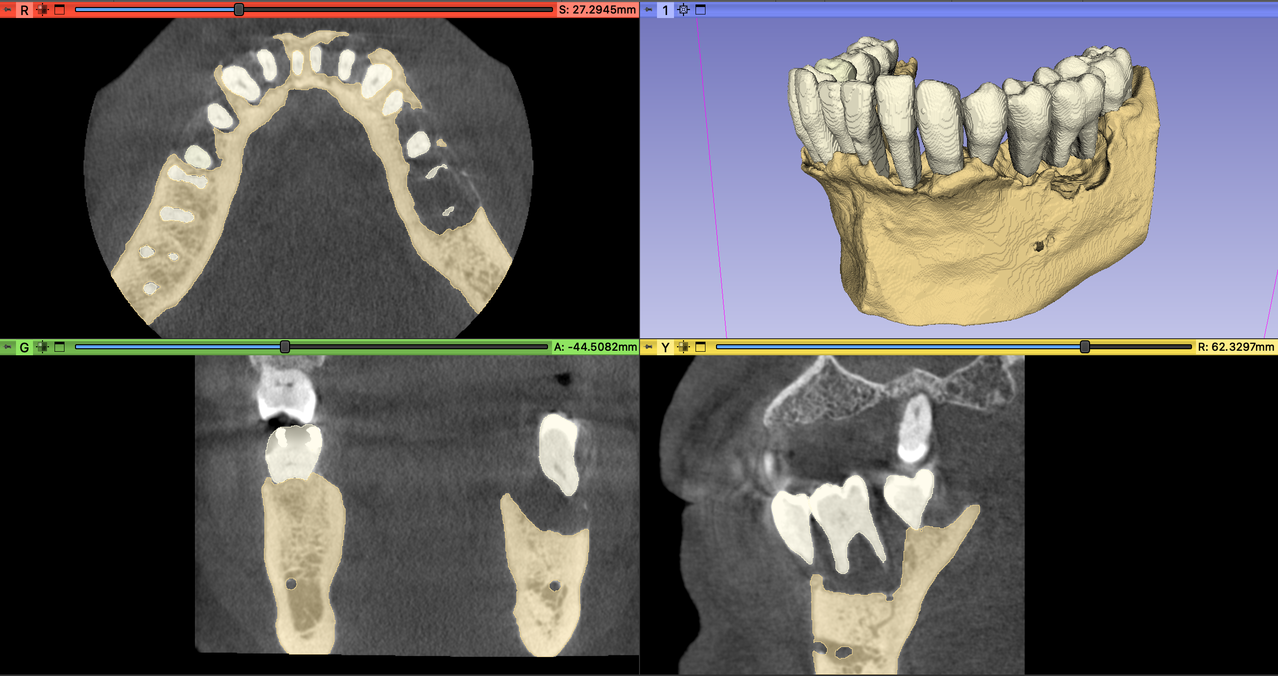
Tooth numbering scheme (EU):
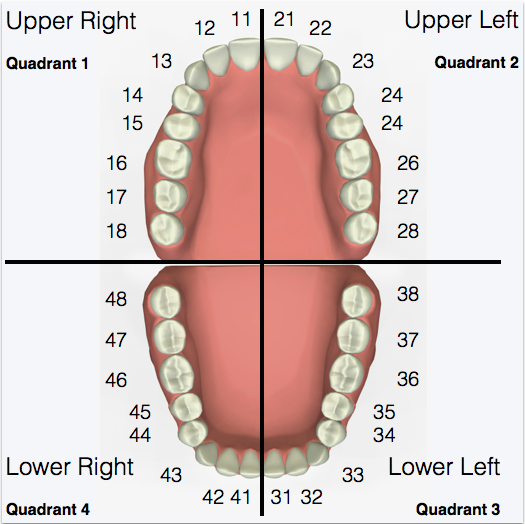
New option in segmentation export to consider terminology:
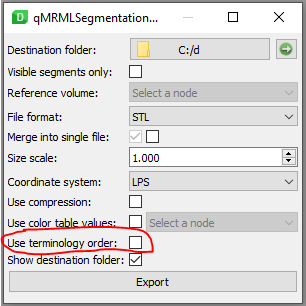
Draft of dental segmentation terminology:
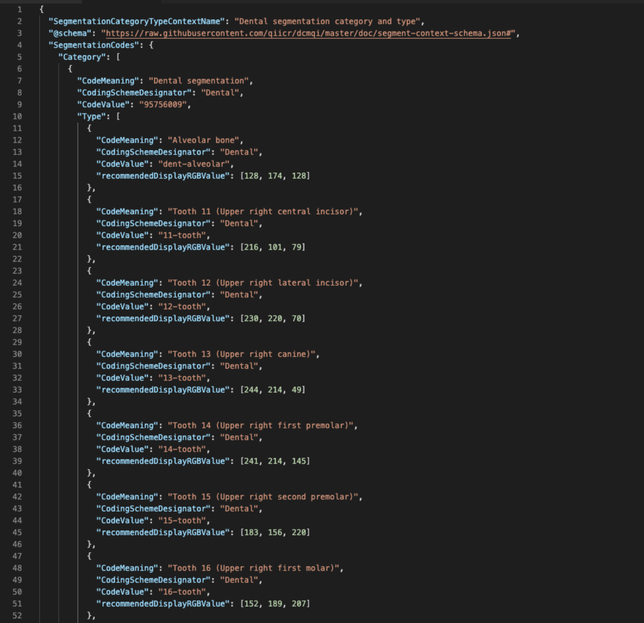
Background and References
- Palkovics D, Mangano FG, Nagy K, Windisch P. (2020) Digital three-dimensional visualization of intrabony periodontal defects for regenerative surgical treatment planning. BMC Oral Health, 20: 351.
- Palkovics D, Pinter C, Bartha F, Molnar B, Windisch P. (2021) CBCT subtraction analysis of 3D changes following alveolar ridge preservation: a case series of 10 patients with a 6-month follow-up. Int J Comput Dent, 24: 241-251.
- Palkovics D, Solyom E, Molnar B, Pinter C, Windisch P. (2021) Digital Hybrid Model Preparation for Virtual Planning of Reconstructive Dentoalveolar Surgical Procedures. J Vis Exp, doi:10.3791/62743.
- Sólyom E, Palkovics D, Pintér C, Mangano FG, Windisch P. (2021) Virtuális tervezés és volumetrikus kiértékelés egy komplex parodontális defektus regeneratív-rekonstruktív sebészi ellátásában: Egy eset bemutatása. Fogorvosi Szemle, 114: 120-130